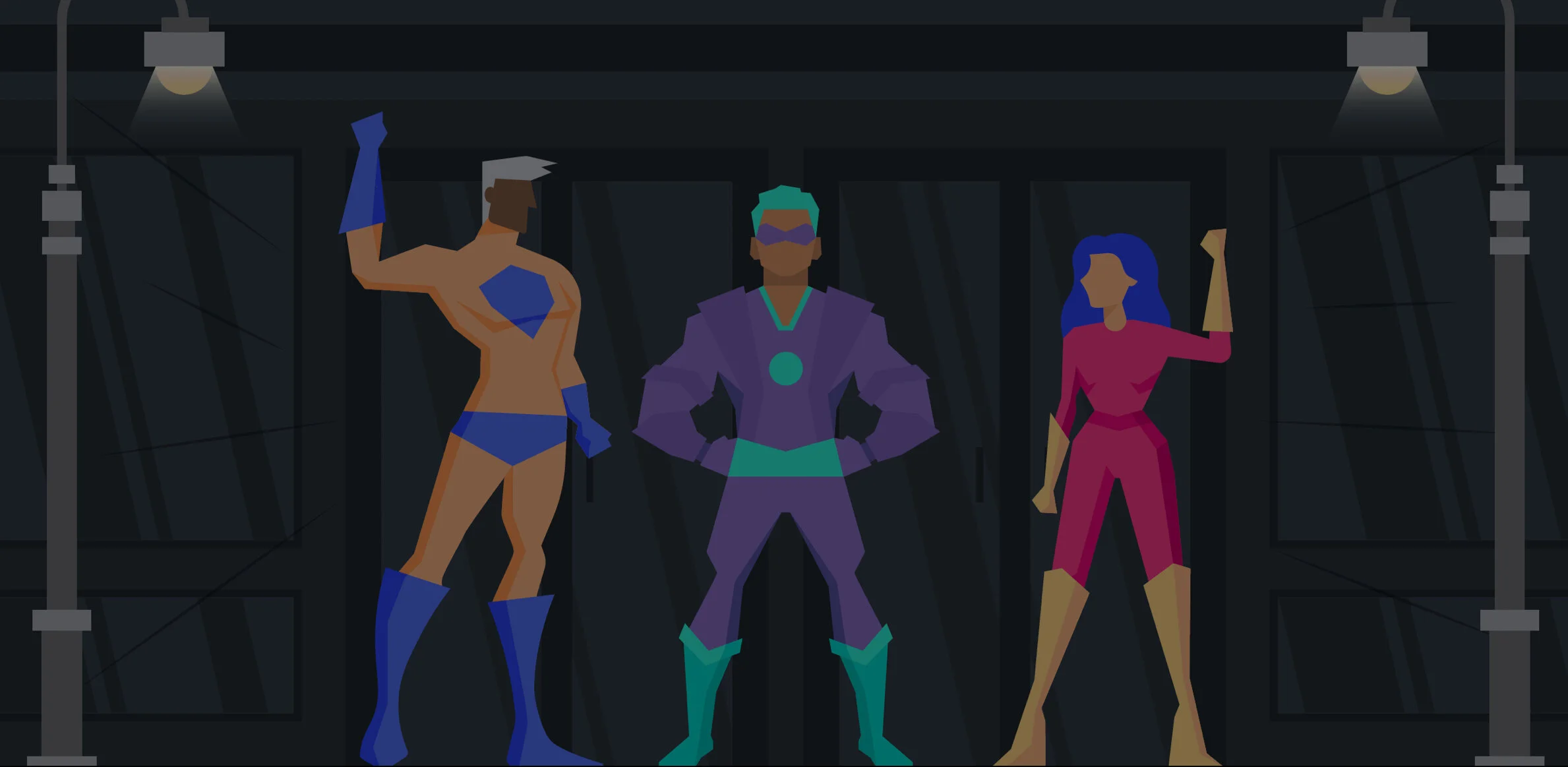
Marketo Premium Events
Premium Events are Event programs on steroids. An event marketer's main job to be done at the end of the day is increase the conversions and artificial intelligence was there to lend a helping hand for them to get there
Role: UX Designer
Location: Marketo HQ
Duration: 13 months
Tools: Milanote, UsabilityHub, Balsamiq, Sketch, Principle, Invision
Background
Marketo started dabbling in the oceans of Artificial Intelligence back in early 2018 with a proof of concept feature in collaboration with the Google Cloud Platform, then branded as Marketo's Audience AI. After receiving some pretty positive feedback from the users and customers alike, Marketo took a short break before realigning their narrative around the AI journey and kickstarted Premium Events. We introduced this feature as an add-on, for event marketers, and I was only involved until a few rounds of beta for the project and some partial GA launches.
Problem description
We as the product experience team realized that an event marketer's main job at the end of the day is to increase conversions. And we wanted to try and help them reach that goal. The main route we thought appropriate was for an event marketer to run super targeted event campaigns, set goals, and then track and try to meet those goals. If they could also get a more finely painted picture about the registrants and attendees of their events, they could understand their audience that much better to run the event and can also help sales team with their conversions.
Design process overview
We heavily relied on research to iterate on the initial fuzzily defined requirements and then fine tune our vision of the feature. What followed was a series of ideation and iterations, validating those concepts and then putting the features in front of beta customers.
1. Generative research
This was one of Marketo's first projects that was driven by generative research from the very beginning. Our main goals:
Discover user needs around an event marketing
Understand the user journey in a day of an event marketer
Generate concepts, test them, and validate the user requirements
Identify the most relevant features and prioritize based on applicability to a larger user base
127 Survey Responses
To start with we posted a survey on Marketo's community which not only provided us with a ton of information to get started but also helped us filter our users into potential interviewees
11 Interviews
Later we interviewed users to map a user journey. We also introduced a few concepts as we progressed along the interview process to start forming initial feature ideas and test them early.
Synthesis from the research
Principles
Marketo had never offered any real intelligence for the users to incorporate into their workflows. So, it was important to identify some some guiding principles, ground rules if you may, to build and foster trust with the users.
Augment not automate
AI should serve as a helping hand and not take over their day-to-day. Because, not only is AI not that smart yet, but also people don't fully trust it.
Explain when necessary
For AI to seamlessly mesh with the current workflows, it needs to be trusted. Explainability thus becomes a very important aspect to build trust.
Configurability is key
Every organization, every team operates differently.Thus, the marketer should be able to configure the models and KPIs as they see fit.
2. Ideate. Conceptualize. Test
This is one of my favorite stages in any process where you get to explore as many options as you'd like and let that creativity to really flow, no constraints and limitations to fixate on, just conceptualizing!
The initial concepts revolved mainly around how we could infuse the AI pieces not only into the marketers workflow within Marketo but also to fit in their marketing timelines. We figured that contextual and timely recommendations would compliment all the features greatly.
Predictions in filtering logic to build the target audience was sought after as a potential feature
The other place we saw as an opportunity to supplement with AI were Event Reports. Giving a way to track KPIs and get insights in these reports could help big time
3.a. Problems to solve for
After the initial synthesis of the research and concept testing we started to get a clearer idea of what we should design and build as the product requirement started to take a more concrete shape. We decided to target these problem areas to begin with:
Whom to target?
The users run often massive campaigns casting wide nets hoping to catch any lead they can - a lot of guesswork involved
How to track and meet goals?
Not knowing whom to target is more hurting when you don’t have an easy way to set and track goals for KPIs, or take actions to meet a set goal
Who really is my audience?
When you do have registrants coming in, how to cater an event towards the incoming audience becomes difficult if you don’t know them
3.b. Define features and success metrics
To solve for the problems we laid down to be solved, we were aiming to release some initial core capabilities and we outlined what success would mean for this package.
Predictive Filtering
Building blocks for users to incorporate which helps them filter better and run more targeted campaigns.
Goal setting + tracking
A way by which the users can set goals for their conversions and a dashboard to monitor the progress
Predictions and Insights
Projections about their audience and programs progress to help them meet goals and know their leads
🎯 Lift of at least 1.5x in their conversion rates
🎯 Adoption in at least 3/5 event programs
🎯 An accuracy rate of around 60% for SMB customers
Apart from the key feature metrics, we also had new usability metrics courtesy of different strategies for Marketo’s platform as a whole.
Event Programs scored a 3.53 on meeting needs and 3.31 on ease of use on a scale of 5 in Marketo’s Classic Platform. New features in Sky Event Programs had to match at least those numbers
4. Finalize features and mid-fidelity testing
Upon validating our initial product requirements, we began to shape up our vision of the product in the form of some mid-fidelity designs. User testing of the mid-fidelity designs also shed some light on their flaws
Predictive Filters
A way for marketers to find people most likely to convert. Also, a filter to help them look for lookalikes to other audiences, expanding the net but not throwing it out in the dark.
We went back and forth over the controls of the filter inputs, but left it as an open field at the end.
During testing we realized open fields would make the most sense since the marketers initially would play around to see what leads are being qualified by each value input before finalizing on one.
Although the less advanced users wanted to stick to categorical input, looking at how Marketo at the core is pro-flexible, it wouldn’t play well to confine the input choices.
Goal Setting and Tracking
A way for marketers to set and track goals for success statuses, and get predictions and recommendations to meet the goals
We made that front and central of reporting page. Predictions and recommendation for each of goals separately would show up, with data supporting the insights
We heard a range of feedback around the visuals and the information consumption. The pieces that stood out were:
Predictions weren’t well represented given so much information being surfaced
The actions on the ‘additional people found’ wasn’t always just ‘Send’ an invite. But more to cherry pick from the list to send the invites
The other interesting piece of research that we came across by Adobe Research team (post acquisition) was this statistical distribution of things users think are important when it comes to AI - understand and validate.
5. Iterate. Test. Repeat
As is after any testing, we went back to iterate with the new findings and old gumption. With the influx of new data points to consider from the AI backend too, this was a grit-testing phase. Iterating as best as we could with all the limitations and technical constraints weighing in on decisions.
A lot of discussions revolved around how to show a breakdown of the likelihoods for leads in addition to the goal tracking piece We were getting additional insights from the backend that could supplement information regarding who in the remaining audience are highly or less likely to convert
We focused quite a bit on different ways of representing the breakdown and goal tracking.
Considering myriad different data visualization approaches was the hallmark of this iteration stage
We also iterated on ways we could suggest more people to the marketer to invite incase they aren’t meeting their goals per predictions.
In line with exposing supporting data we wanted to show why these ‘lookalikes’ are suggested.
The other place in product we surfaced predictions at a lead level was in the Members page of an event program. Here the users could then sort, filter, and take action based on the likelihood of a lead
Audience insights was another area we introduced that could show the user a true picture of who their audience really is, what there are interested in and where they are coming in from to help their organization with attribution
6. Beta Implementation
After running into hundreds of roadblocks, complications, and errors and issues with all AI models, we currently have an open beta running (a closed beta was tested with 6 users prior). We are continuously gathering feedback and data and trying and learn from it.
The beta version of predictive filters has an additional logical input to constraint certain cases.
We also introduced contextual insights to help them draw conclusions based on past data
🏮Also realized, it is not easy to design, build, and then maintain new features atop an aged system. Every step needs to be carefully thought out to not land in a hot pool of mess.
Predictions for goal tracking took a severe hit due to underperforming models, so to maintain users' trust with the accurately performing models we replaced the predictions with 'Estimations' which are based off of past similar programs.
That coupled with data about past programs was proving useful as was reaffirmed by a recent card sorting exercise.
⚖ We sat down with huge excel sheets of data from the beta customers to actually make sense of what calculations can be helpful.
working on excel sheets also helped us come up with insight messages about user’s past performances to reaffirm users' trust in the AI features, since the models that were producing meaningful results were exceptionally accurate.
We also hid the absolute likelihood values behind simple data visualization at a lead level in a grid. The main purpose of the user here, was quickly gauge how likely the leads are. Absolute values at a lead level provide little value since they are too granular to consume
Next Steps
These beta features went global in February 2020. With constant feedback and learnings from the beta users, we were constantly making improvements. Some key next steps from as of December 2019 were:
Run usability benchmarking test to see how the scores line up against Classic platform benchmarks
Prioritize the various data points in results tab by rank sorting the results of a card sort exercise
Monitor the conversions and performance results to meet the success metrics
Iterate on ‘New Lookalike’ recommendations as the AI/ML model gets ready behind the hood
Choreograph the notifications time and flow based on the research findings on event timelines
Key Takeaways
Working on this project for over a year (or probably more) taught me a great deal. I realized that only 49% of a designer's job entails designing, the rest 51% is to deal with people. Talk, research and deal with stakeholders of all kinds. Setting and managing expectations was one of the most important skills that I got introduced to (still learning the tricks of the trade). B2B features can be exhaustive, and an absence of streamlined processes can greatly affect your sanity. This project truly schooled me more on the operational and communicative side of UX, and I continue to be a better designer thanks to this. Working closely with the product managers (and borrowing that hat every now and then to run operations) has been enlightening to learn about how to give and take, and compromise with engineering capabilities to keep the business sides of things running smoothly.